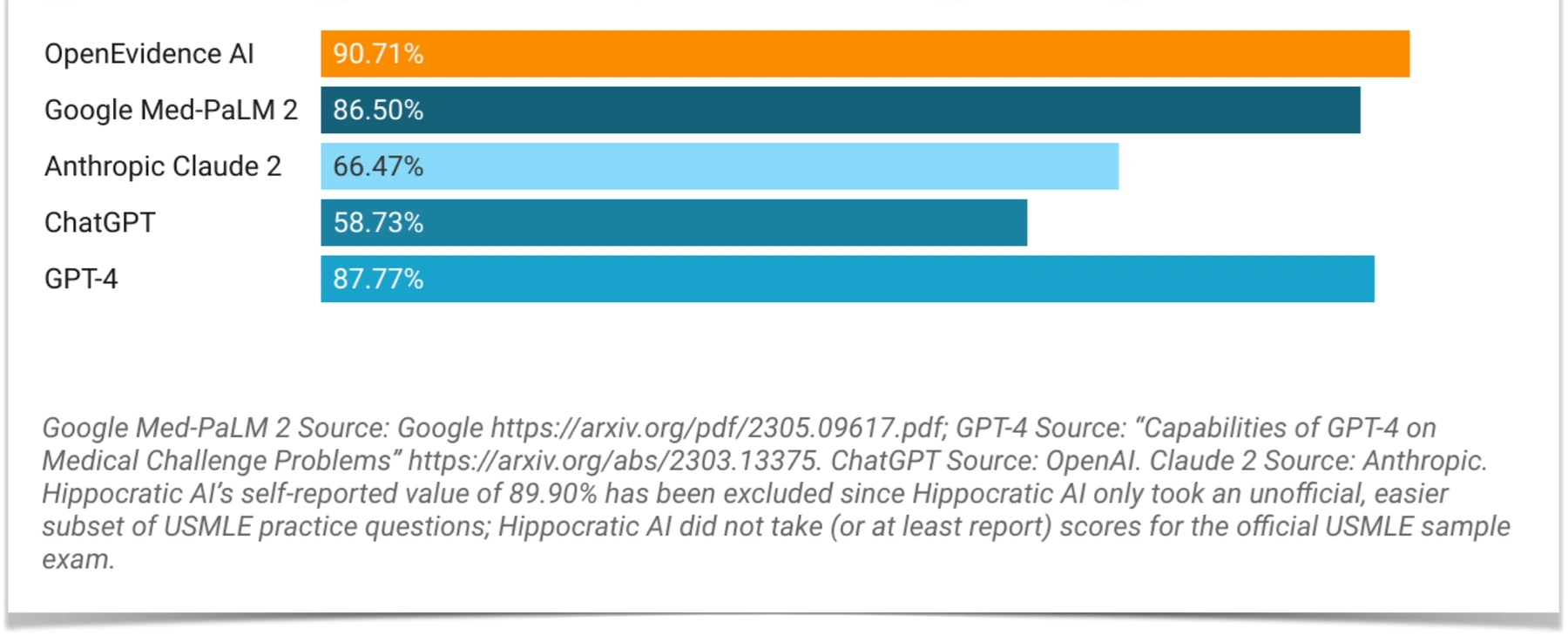
OpenEvidence AI, the large language model-based artificial intelligence powering the automatic ingestion, analysis, synthesis, and research on openevidence.com, has reached a new milestone as the first AI to score over 90% on the United States Medical Licensing Examination (USMLE). OpenEvidence makes 77% fewer errors on the US Medical Licensing Exam than ChatGPT, 24% fewer errors than GPT-4, and 31% fewer errors than Google’s Med-PaLM 2. This result further demonstrates the immense potential that AI and OpenEvidence hold in revolutionizing healthcare, as the score achieved surpasses "expert-level performance".[1]
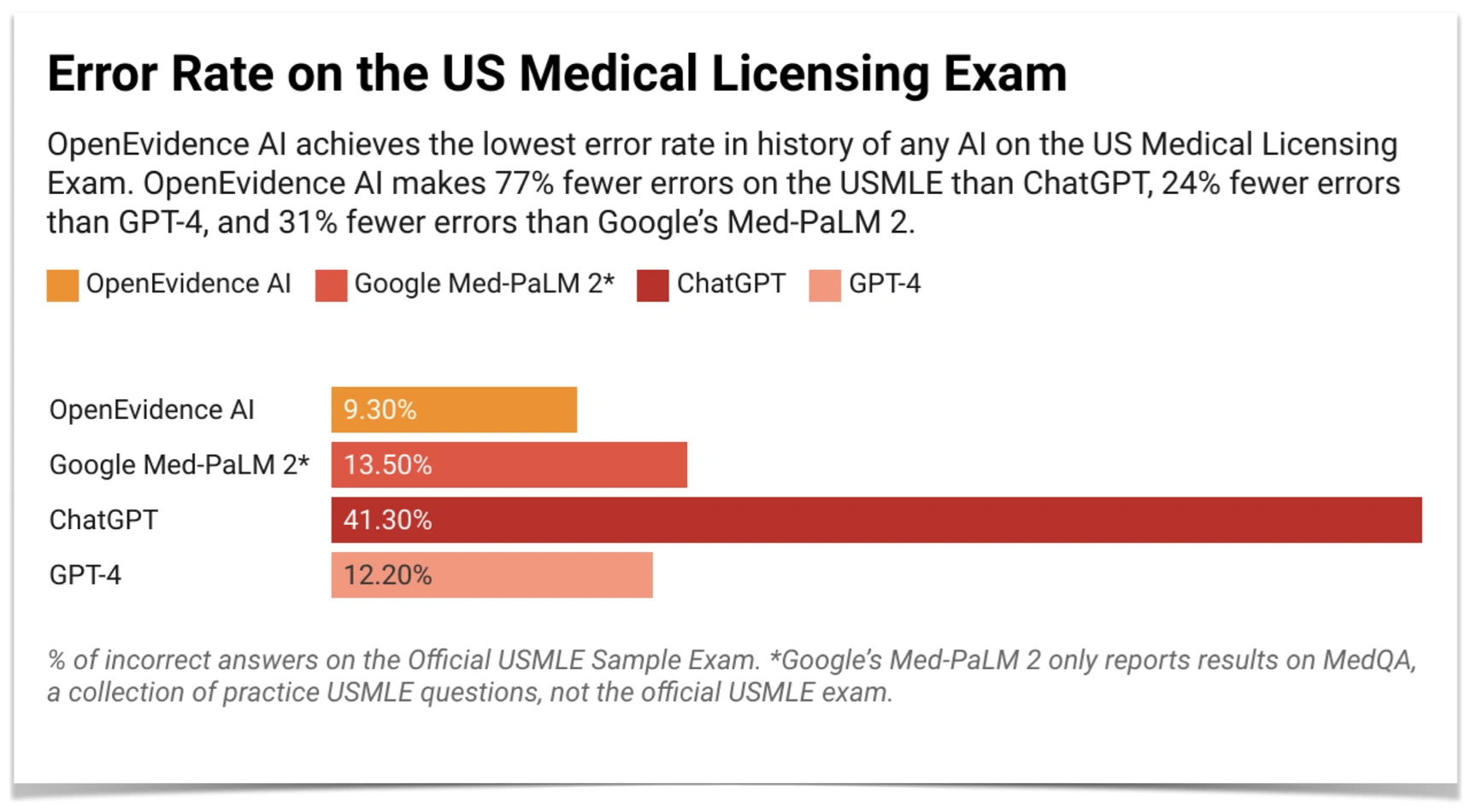
The USMLE is a three-step examination for medical licensure in the United States. It assesses a physician's ability to apply knowledge, concepts, and principles, as well as demonstrate fundamental patient-centered skills that form the foundation of safe and effective patient care. Traditionally, the USMLE has been a rigorous examination that demands a broad understanding of biomedical and clinical sciences, testing not only factual recall, but also decision-making ability. Artificial Intelligence achieving a score above 90% on the USMLE—a feat almost unthinkable even 18 months ago—showcases the tremendous strides that Artificial Intelligence generally—and OpenEvidence specifically—have made in understanding and applying complex medical concepts.
To measure the performance of our model on the USMLE, we use the official sample exam from 2022. We believe that this is the most authentic measure of performance on the USMLE. A sample question from the USMLE Step 3 sample exam is shown below:
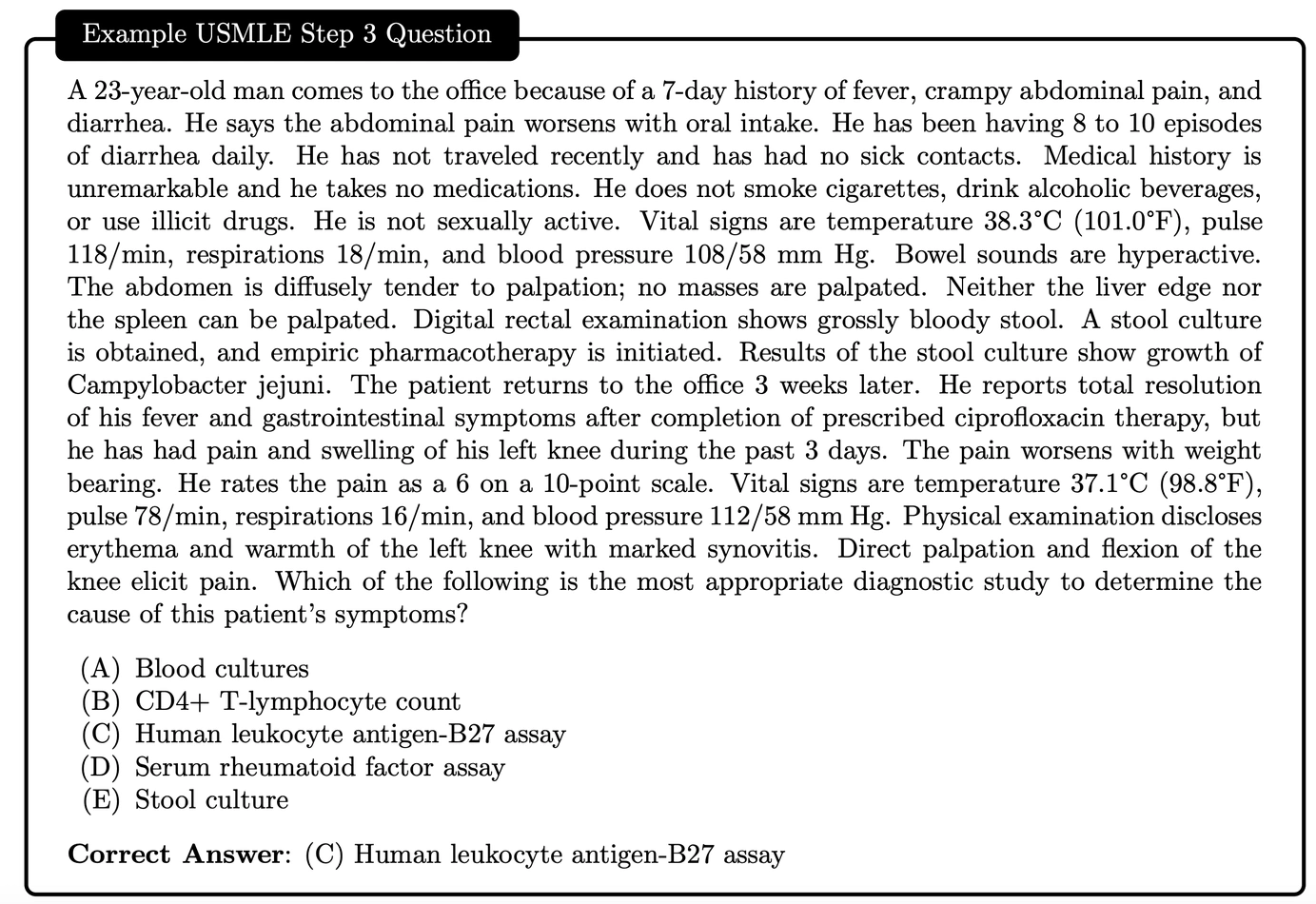
As of July 11th, 2023, both GPT-4 and ChatGPT both incorrectly answer (A) Blood cultures, whereas OpenEvidence’s AI correctly answers (C) Human leukocyte antigen-B27 assay.
The USMLE consists of 3 different exams that medical students will take at varying points in their career.
The Step 1 exam is generally taken after the second year of medical school – it covers anatomy, biochemistry, physiology, microbiology, pathology, pharmacology, and behavioral sciences. The test focuses on principles and mechanisms underlying health, disease, and modes of therapy.
The Step 2 exam, which is generally taken during the 3rd or 4th year of medical school, consists of two different parts: Clinical Knowledge (CK) and Clinical Skills (CS). Generally speaking, the Step 2 CK test assesses medical knowledge and understanding of clinical science necessary for the provision of patient care under supervision. It involves disease diagnosis and management. Unfortunately, the Clinical Skills (CS) portion of the USMLE is a practical exam, meaning we are not able to test the model on it.
The Step 3 exam, which is generally taken after the first year of residency, attempts to assess whether a graduate can apply medical knowledge and understanding of biomedical and clinical science essential for the unsupervised practice of medicine. The examination includes patient management, diagnosis, prognosis, and understanding of pathophysiology of disease.
Below, we show the performance of OpenEvidence’s AI system per Step exam, relative to ChatGPT.
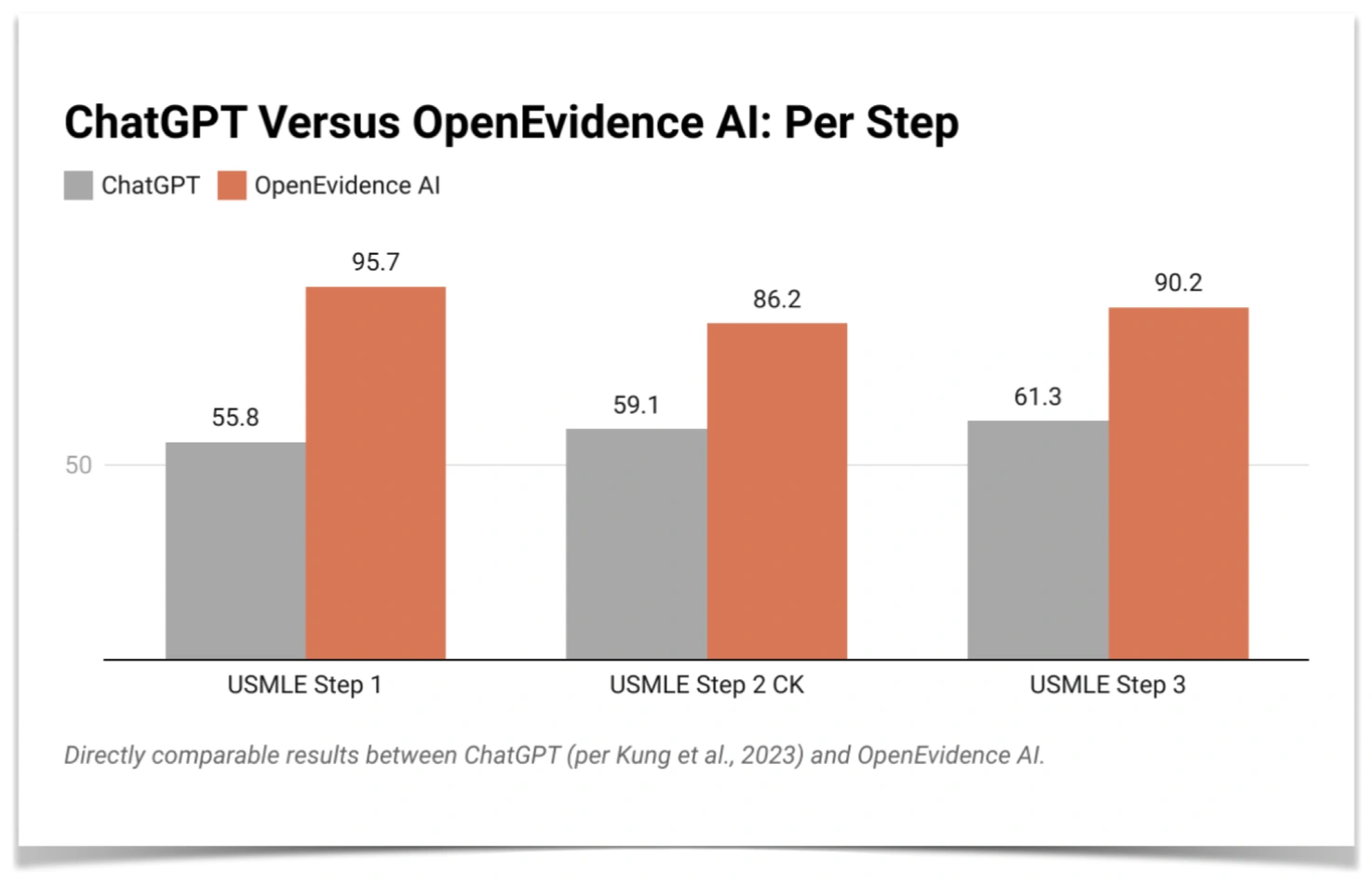
To measure the performance of our model on the USMLE, we use the official sample exam available at usmle.org. We do not run on questions that contain images. This has established precedent, as we use the exact set of questions as detailed by Kung et al., 2023. This results in 94 questions from Step 1, 109 questions from Step 2, and 122 questions from Step 3 (325 total). We use the spreadsheets provided by Kung et al., 2023, but fix a recording error with Question #78 from the Step 3 spreadsheet.[2]
For ChatGPT based results reported, we use the numbers reported by Kung et al., 2023. For GPT-4 based results, we report results from Nori et al., 2023. Nori et al., 2023 does not report per Step scores on the text-based portion of the USMLE – they only report their average performance.
We are unable to directly compare to Google’s MedPALM-2, as Google only reports their results on USMLE style questions (MedQA), and not the official sample exam. MedQA is a set of scraped practice USMLE questions. The dataset has multiple differences. First, the scraped dataset consistently contains 4 options per question – the official sample exams tend to have 5 options per question. The dataset originally contained more answers, however these were removed by the original paper (Jin et al., 2019) in an attempt to avoid copyright infringement.
We additionally found in a cursory analysis that roughly half the questions were labeled as practice questions from Step 1 and that there was no distinction between Step 2 and 3 questions. This could mean that a disproportionately strong performance on Step 1 questions could skew performance. Lastly, it's unclear that the types of questions in MedQA appear at the same frequency as the official sample exam. For example, it's possible that MedQA has a disproportionate amount of questions about a particular topic. Further analysis is needed to determine how performance on MedQA relates to the true performance on the USMLE.
Another resource that has been used to measure performance of AI on the USMLE are the self-assessment exams. These are a set of practice USMLE tests from the National Board of Medical Examiners. Unfortunately, these tests are hidden behind a $900 paywall, which would disadvantage independent researchers with limited funding when comparing their results. Further, these tests appear to be easier than the official sample exam – Nori et al., 2023 reports that GPT-4 scores +2% higher (14% lower error rate) on the self-assessment than the official sample exam. This may be due to the fact that the official USMLE sample exam is designed by a committee of physicians that ensure that the style, content, and format are all directly aligned with the USMLE's actual evaluation – this may not be true for the self-assessment practice tests. For these reasons, we exclusively run on the official sample exam.